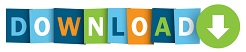
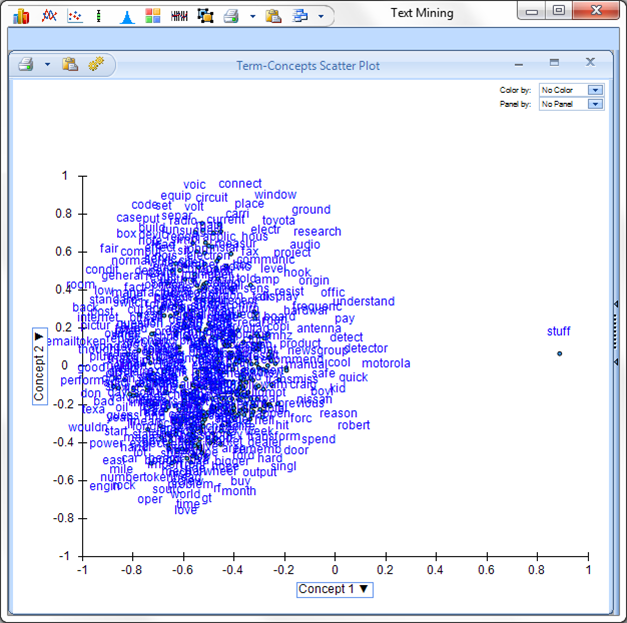
Thus, it is not proved that the presented structure can indeed exploit nonlinear waves to achieve better performance in problems beyond linear signal processing.
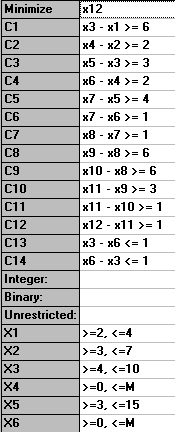
It is admitted that numerical simulations with the computational learning machine do not fully support the premise of the study, as the RNN-equivalent nonlinear structure shows similar performance to what is achievable by linear propagation. 2 is an original, fresh approach to wave-based computing, but leaves crucial questions unanswered. Training of the neural network is implemented by adjusting the spatially dependent wave propagation speed by gradient-based computational learning. In their work, the nonlinearity of the medium is modeled by a spatially varying and intensity-dependent wave propagation speed. Specifically, it was shown that if a substrate is described by the nonlinear wave equation and this substrate is excited and probed at given points, then the equations that give the wave dynamics between the prescribed points map to an RNN. 2 presented a theoretical framework for implementing a recurrent neural network (RNN) in a medium described by a nonlinear wave equation. This is where wave-based computing concepts show their strengths: if the computing device is realized in a wave-propagating substrate, then interference patterns realize an all-to-all interconnection between points of this substrate. Stand-alone neuronal units have little utility-there should always be an effective way to interconnect those devices to computing systems. These are the application areas where emerging devices have the potential to show substantial benefits over MOS switches 1.Ī central challenge of the research on neuromorphic devices is that most computing models require highly interconnected systems, i.e., artificial neurons with a large number of connections, often all-to-all connections. A more recent development is that traditional, MOS-transistor-based devices turned out to have a strong staying power for Boolean, digital logic-which has driven the research of emerging nanoelectric devices towards neuromorphic, analog problems. It was realized long ago that digital systems (let they be CPUs or Graphics Processing Units, GPUs) are rather inefficient for such inherently analog tasks. The interest in neuromorphic computing hardware increased significantly in recent years, for two main reasons. We envision small-scale, compact and low-power neural networks that perform their entire function in the spin-wave domain. We show that the behavior of spin waves transitions from linear to nonlinear interference at high intensities and that its computational power greatly increases in the nonlinear regime. A custom-built micromagnetic solver, based on the Pytorch machine learning framework, is used to inverse-design the scatterer. Training the neural network is equivalent to finding the field pattern that realizes the desired input-output mapping.

The interference of the scattered waves creates a mapping between the wave sources and detectors. Weights and interconnections of the network are realized by a magnetic-field pattern that is applied on the spin-wave propagating substrate and scatters the spin waves. We demonstrate the design of a neural network hardware, where all neuromorphic computing functions, including signal routing and nonlinear activation are performed by spin-wave propagation and interference.
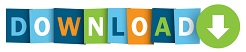